Human-AI Systems /
How Is Human-AI Collaboration Shaping Our World?
The integration of artificial intelligence into professional and personal domains represents an evolving landscape of collaboration between humans and machines. Early optimism focused primarily on augmenting human capabilities, but real-world deployment reveals multilayered complexities. Pioneering researchers such as Ed Hutchins explored distributed cognition in the early 90s, studying AI systems as extensions of human knowledge rather than standalone tools. His theoretical frameworks sparked new waves of human-centered AI research. By the late 2010s, rapid advances in machine learning drove increased autonomy and influence for AI. However, as sociologist Zeynep Tufekci observed, lack of transparency around data-driven systems undermined public trust. AI thought leaders like Joanna Bryson began stressing human oversight and explanation as mandatory counterbalances to growth. Today, HCI practitioners continue navigating tensions between harnessing AI capabilities and maintaining human control. From enhancing personalized education to refining conversational interfaces, integrating intelligence assistance requires meticulous alignment of model capabilities with user needs and values. This delicate collaboration remains an iterative process of calibration. The ideal equilibrium between humans and AI yields relationships where machine analytical skills mesh fluidly with human judgment to produce cohesive, constructive partnerships across multiple facets of life.
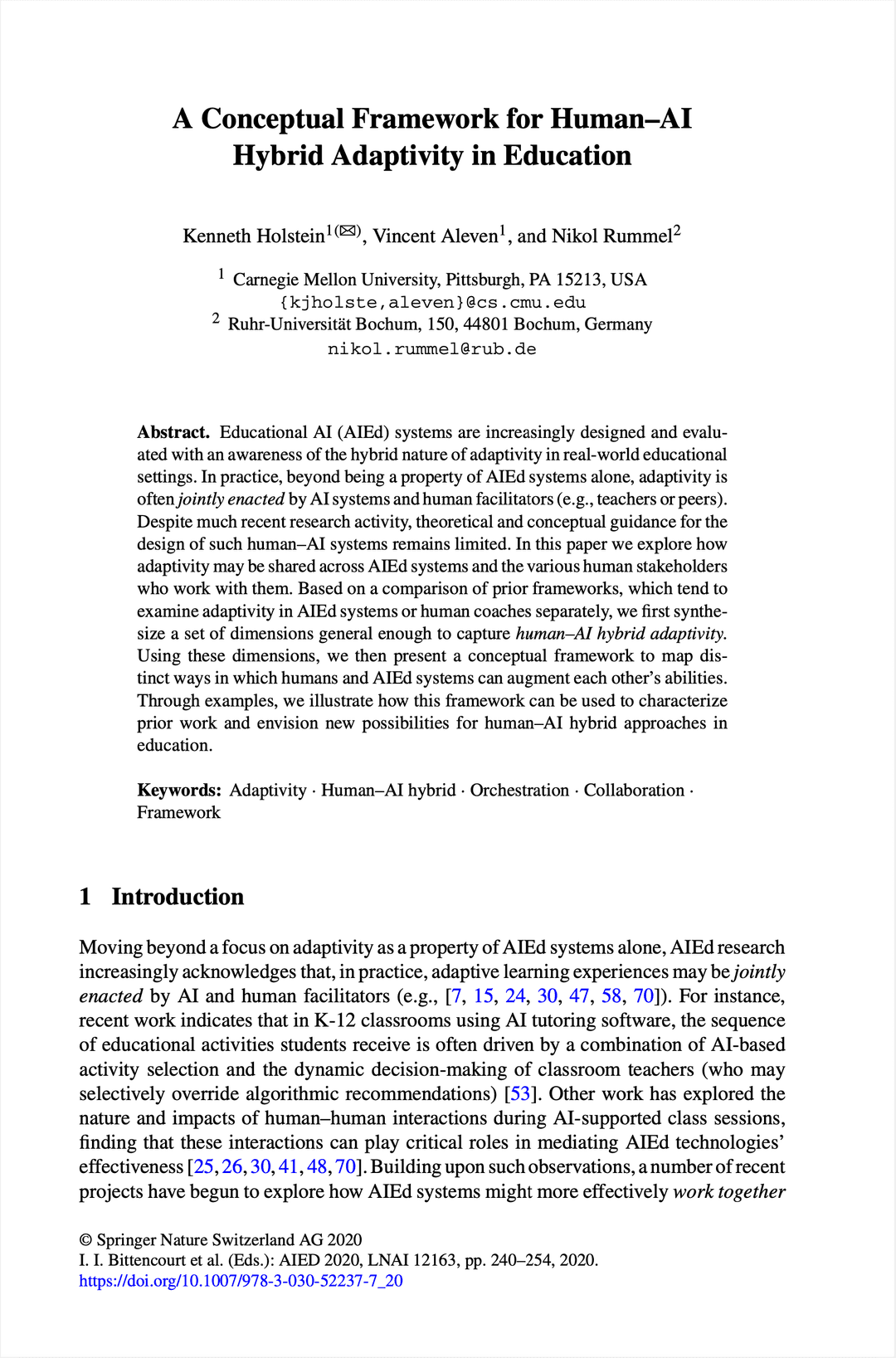
A Conceptual Framework for Human–AI Hybrid Adaptivity in Education
Kenneth Holstein, V. Aleven, N. Rummel · 01/06/2020
This HCI paper introduces a novel conceptual framework for developing adaptive educational systems that leverage AI's predictive capabilities alongside human expertise. This hybrid approach aims to increase adaptivity in learning environments.
- Hybrid Adaptivity: This is a unique combination of AI predictive capabilities with human expertise to create more adaptable learning environments, potentially enhancing student engagement and outcomes.
- AI Benefits: The authors stress that AI can excel in predicting students' learning trajectories and identifying obstacles, thus enabling targeted interventions and support.
- Human Expertise: However, this technology is not deemed sufficient on its own. Human expertise in instructional design, pedagogy, and assessment are crucial inputs to ensure learning experiences are not only predictive but also constructively engaging.
- Iterative Framework: The proposed conceptual framework focuses on iterative designing, implementing, and evaluating of hybrid adaptive systems, with the involvement of educators and learners in the process.
Impact and Limitations: The paper presents a potential breakthrough for the HCI field, shifting focus from AI-first to an AI-human hybrid educational model. This approach can optimize learning outcomes, while maintaining the human touch that's fundamental in education. However, the paper lacks empirical evaluations, necessitating further research to validate this framework's efficacy in real-world settings.
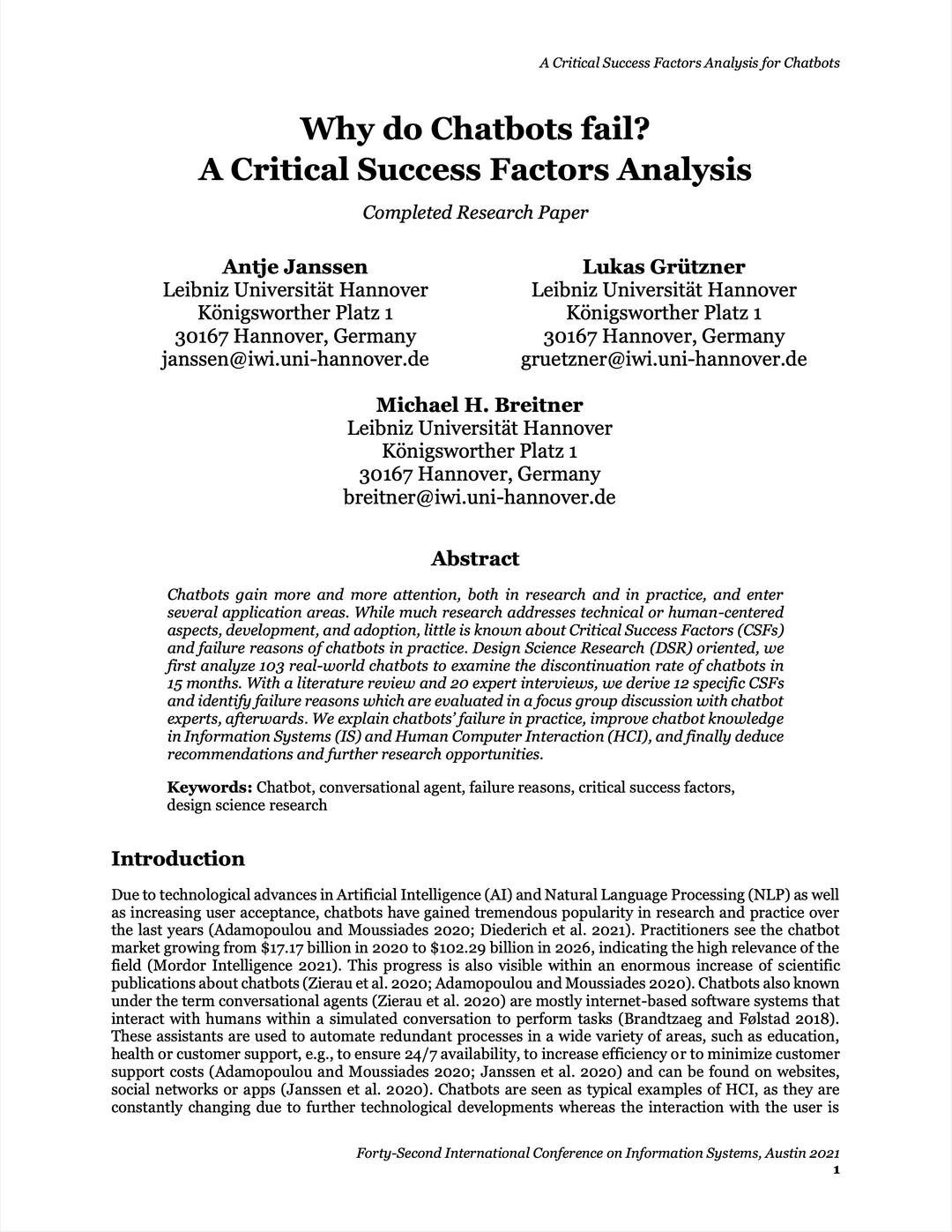
Why do Chatbots fail? A Critical Success Factors Analysis
Antje Janssen, Lukas Grützner, Michael H. Breitner · 01/12/2021
This paper focuses comprehensively on analyzing CSFs (Critical Success Factors) influencing the user acceptance of chatbots in the field of HCI (Human-Computer Interaction). Using an extensive, methodical literature review, the authors expose four key factors significantly affecting chatbot adoption.
- User Expectations: The authors note that unrealistic or incorrect user expectations about chatbot abilities can unduly influence acceptance. They recommend precise and careful presentation of chatbot capabilities to users.
- Interaction Quality: They argue that a chatbot’s interaction quality, including conversation flow and usability, directly affects user satisfaction and thereby, acceptance.
- Perceived Usefulness and Ease of Use: Building on the Technology Acceptance Model, the paper underscores perceived usefulness and ease of use as integral factors for chatbot acceptance.
- Chatbot Anthropomorphism: The degree to which a chatbot appears human-like was found to have mixed implications on user acceptance, making its role ambiguous.
Impact and Limitations: This investigation deepens the understanding of chatbot success factors, assisting developers in creating more user-friendly and widely accepted chatbots. However, the authors acknowledge that there may be other chatbot-specific factors not yet fully explored in this research. They recommend further exploration and user behavioral studies in varied contexts and user groups to gain more nuanced insights.
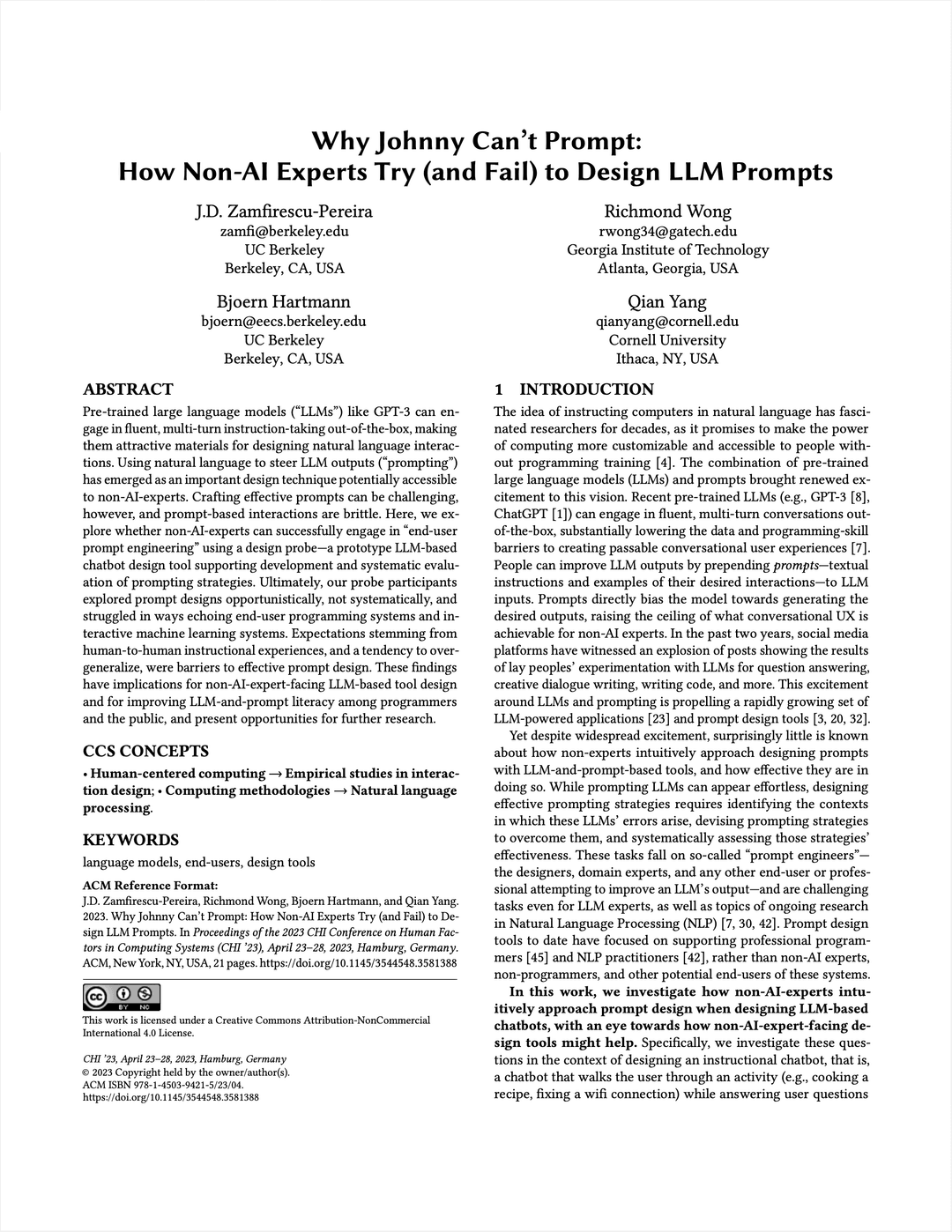
Why Johnny Can’t Prompt: How Non-AI Experts Try (and Fail) to Design LLM Prompts
J.D. Zamfirescu-Pereira, Richmond Wong, Bjoern Hartmann, Qiang Yang · 01/04/2023
This paper investigates the difficulties faced by non-AI experts in designing effective prompts for Language Learning Models (LLMs). This user study uncovered key errors and established strategies to assist non-expert users.
- Non-Expert Misconceptions: Non-experts often hold inaccurate mental models of how LLMs work, leading to ineffective prompt designs. Clarifying these misconceptions can increase prompt effectiveness.
- Prompting Strategies: The authors delineate several effective strategies such as asking explicit instructions, multi-turn prompts, and breaking down complex prompts.
- LLM Limitations Awareness: Non-experts often overestimate LLM capabilities. Enhancing users' understanding of model limitations can guide towards more effective prompts.
- Tool Limitations: Current tools do not sufficiently support non-experts in prompt design. User-friendly interfaces equipped with guidance and feedback mechanisms are much needed.
Impact and Limitations: Developing strategies and tools for non-experts to better interact with AIs has significant implications for HCI, specifically in democratizing and enhancing AI usability. However, the applicability beyond the selected user group and LLMs remains to be seen. Future research could study other AI systems and user groups to expand these findings and validate the proposed strategies.
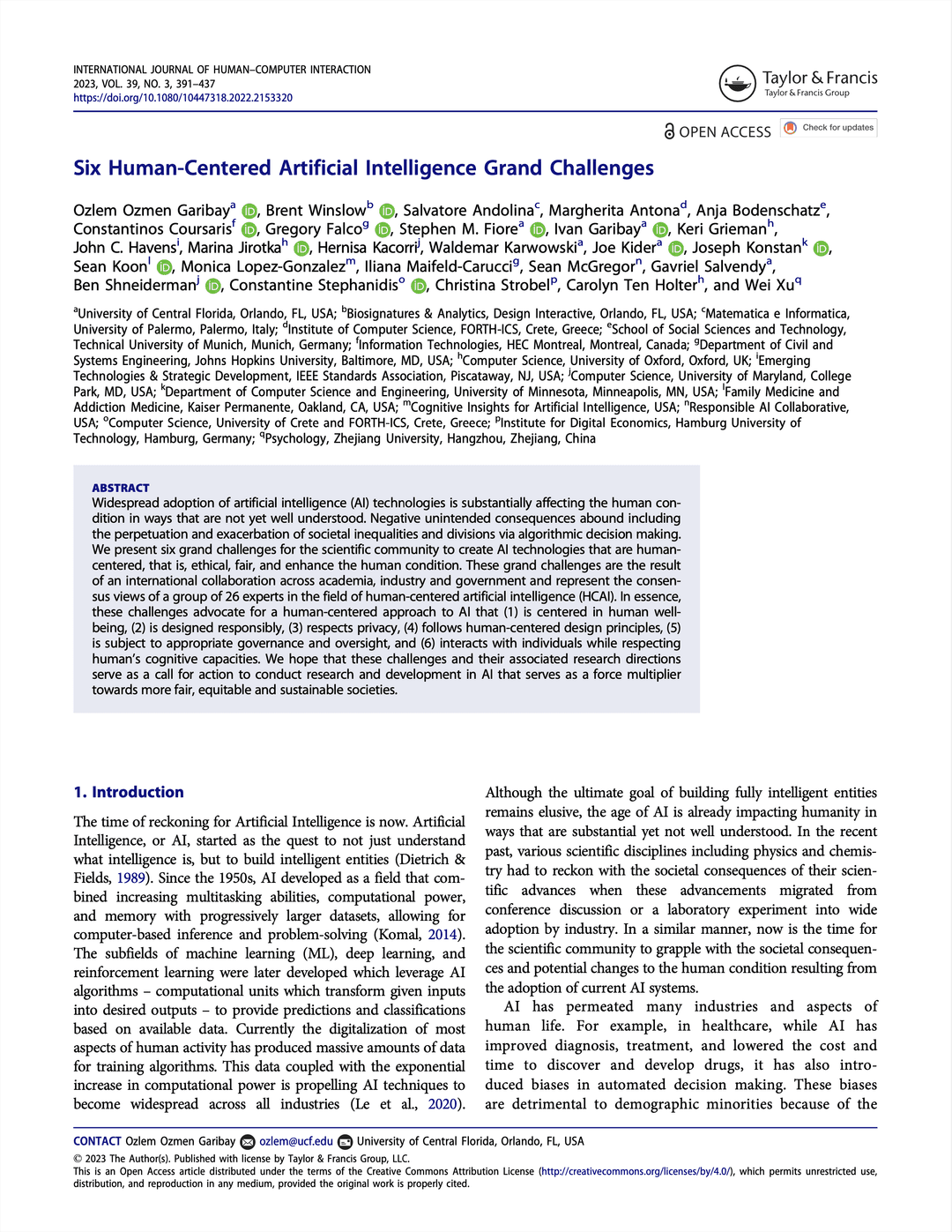
Six Human-Centered Artificial Intelligence Grand Challenges
Ozlem Ozmen Garibay, Brent Winslow, Salvatore Andolina, Margherita Antona, Anja Bodenschatz, Constantinos Coursaris, Gregory Falco, Stephen M. Fiore, Ivan Garibay, Keri Grieman, John C. Havens, Marina Jirotka, Hernisa Kacorri, Waldemar Karwowski, Joe Kider, Joseph Konstan, Sean Koon, Monica López-González, Illiana Maifeld-Carucci, Sean McGregor, Gavriel Salvendy, Ben Shneiderman, Constantine Stephanidis, Christina Strobel, Carolyn Ten Holter, Wei Xu · 01/01/2023
The paper addresses the grand challenges emerging from the intersection of Artificial Intelligence (AI) and Human-Computer Interaction (HCI). By examining various areas of HCI, the authors highlight six key challenges that need to be tackled to ensure a human-centered AI.
- Fairness in AI: AI should be designed to ensure fairness in decisions and accessibility, reducing biases inherent to data collection and processing.
- AI Transparency: The authors argue for enhanced transparency in AI systems, emphasizing the importance of understandability of AI actions for building user trust.
- AI Adaptability: AI should be programmable and adaptable, capable of learning and changing according to individual users' preferences without compromising its usefulness.
- AI Privacy: Data protection is critical in the AI-HCI paradigm, considering the extensive amount of user data gathered and analyzed by AI systems.
Impact and Limitations: The identified challenges directly impact how AI systems are designed, developed, and deployed. Addressing these challenges can lead to more human-centered, fair, and accountable AI systems. However, the authors note some limitations, such as cultural specificities not being considered. Future research should focus on the practical ways of tackling these challenges, potentially offering a transformation in the field of AI-HCI.
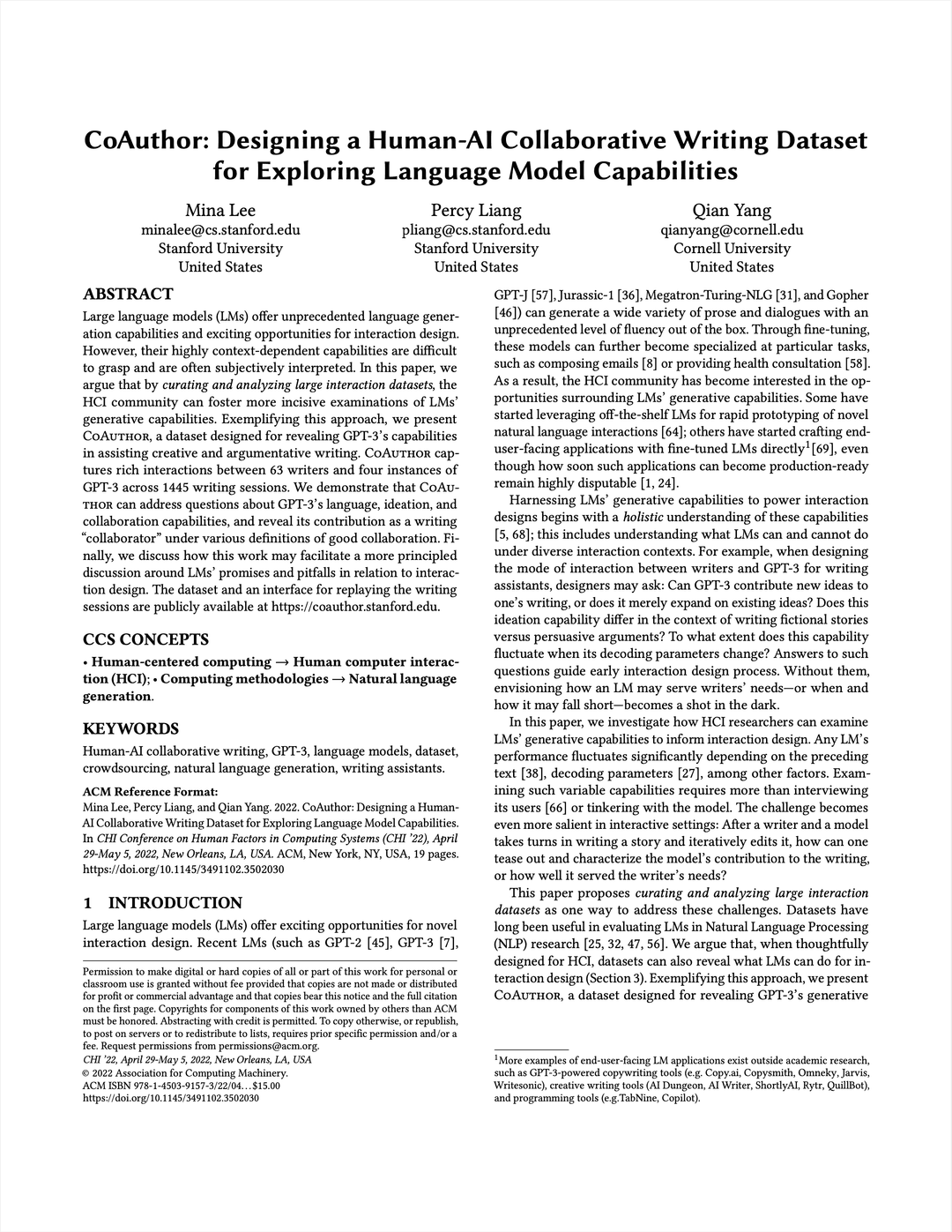
CoAuthor: Designing a Human-AI Collaborative Writing Dataset for Exploring Language Model Capabilities
Mina Lee, Percy Liang, Qian Yang · 01/01/2022
This paper discusses the potential of Human-AI collaboration in the context of creating a collaborative writing dataset, exploring algorithmic capabilities and potential workflows. It presents groundbreaking findings in the interdisciplinary field of HCI and machine learning.
- Human-AI Collaboration: The study underscores the potential of AI as a collaborative tool in creative endeavours like writing. It discusses the design of interaction frameworks and interfaces for successful collaboration.
- Algorithmic Capabilities: The authors experiment with the capabilities of language models across different writing tasks, revealing insights into the technological sophistication and limitations of AI in context-aware understanding.
- Interactive Machine Learning (IML): The research demonstrates an innovative use of IML in the field of collaborative writing. It bridges HCI and Machine Learning, aiding the creation of a more user-friendly, interactive AI environment.
- Workflows: Based on observations, it proposes optimized workflows for scenarios when humans and AIs write together, offering clues for future interfacing and system design.
Impact and Limitations: This paper changes our understanding of AI’s role from a mere tool to a collaborator. It guides the design of future Human-AI collaborative systems and suggests improvements to existing AI language models. However, the research is prototype-based and there is room for testing and refinement in real-world contexts. Further investigation into the co-writing process and advances in machine learning could enhance effectiveness and adaptability of AI collaborators.
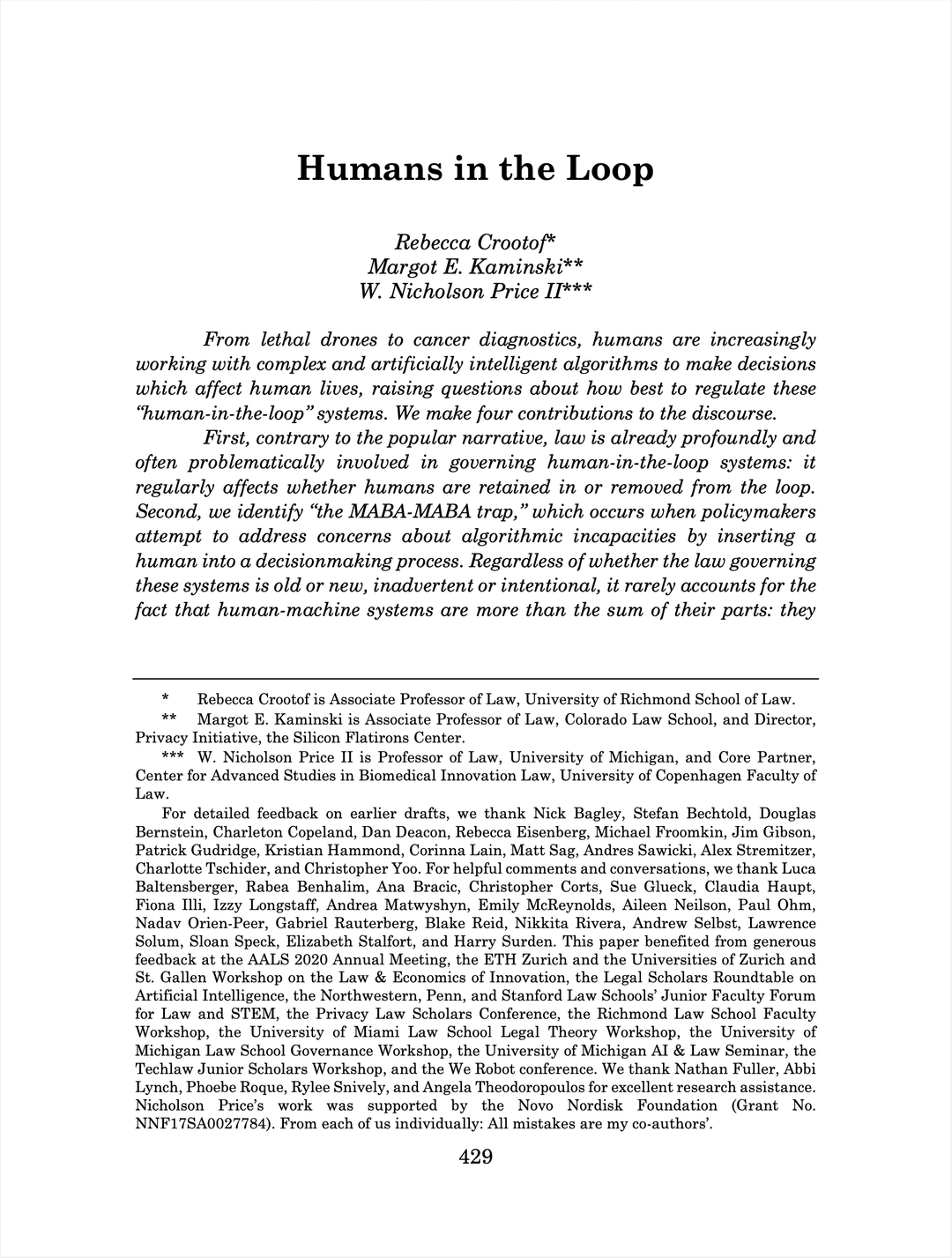
Humans in the Loop
Rebecca Crootof, Margot E. Kaminski, W. Nicholson Price II · 01/03/2023
The paper "Humans in the Loop" explores the role of human intervention and control in computer-driven systems. It uncovers the legal, ethical, and practical dimensions of maintaining a 'human in the loop' in HCI.
- Human Intervention: The authors argue that human intervention enhances ethical decision-making in computer systems by adding a layer of accountability, critical thinking, and empathy, unattainable solely by machine intelligence.
- Legal implications: The discussion considers the legal responsibilities of the human operators in computer systems. For instance, who bears the responsibility when an AI makes a fatal decision?
- Practical Dimensions: It elaborates the practical applicability of placing humans in control at varying degrees across different sectors, thus ensuring the optimal utilization of both human and machine capabilities.
- Bias Mitigation: This study exposes how humans in the loop can help reduce biases in computer systems by providing checks on prejudiced outcomes that machines alone might perpetuate.
Impact and Limitations: This paper outlines the importance of human intervention in AI-driven systems. The findings suggest that adequate human control over automated systems might be the key to more ethical, legally sound, and practical AI applications. However, the paper lacks a comprehensive exploration of the requirements for the effective functioning of 'Human in the Loop' systems, bringing to light a potential area for future research.
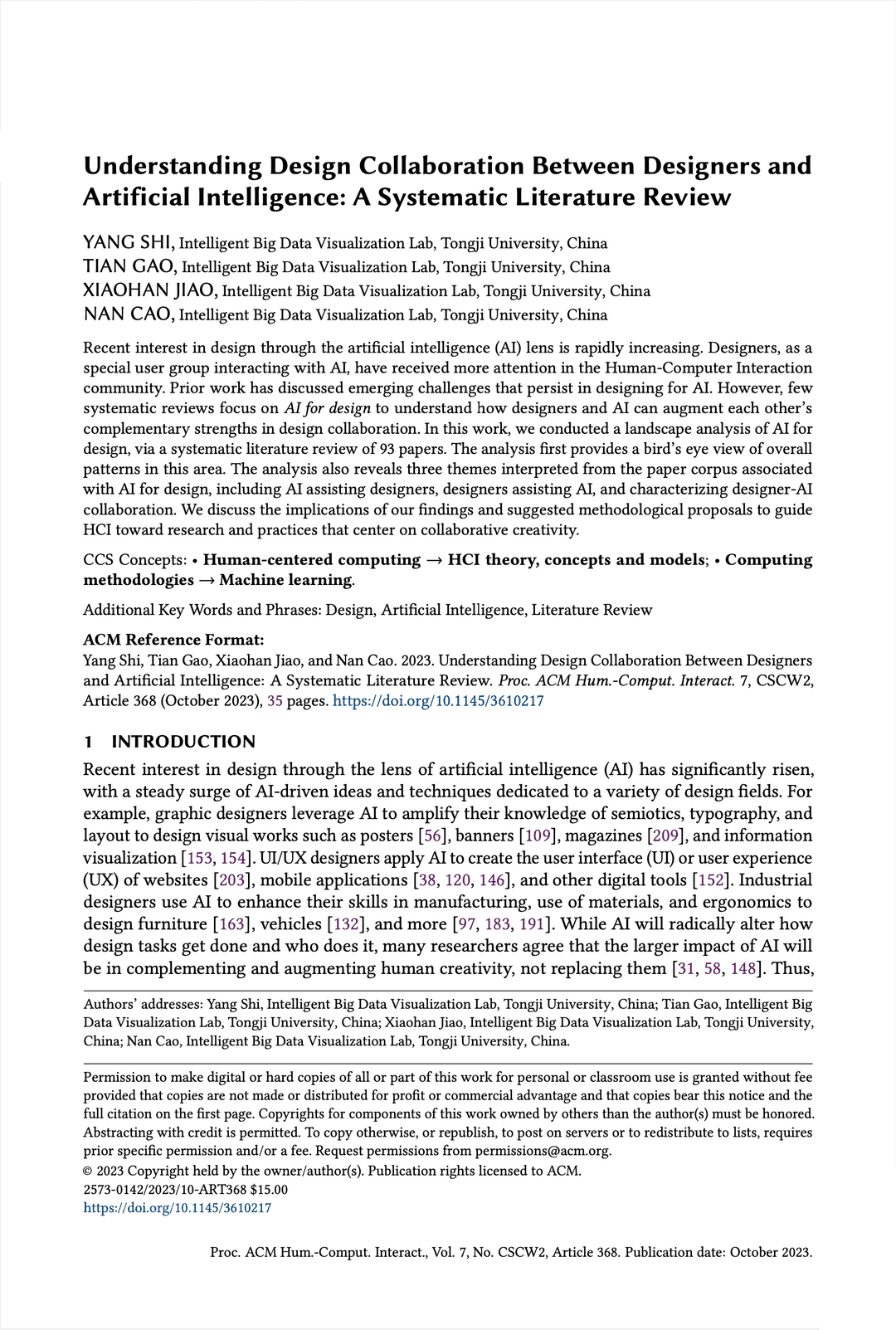
Understanding Design Collaboration Between Designers and Artificial Intelligence: A Systematic Literature Review
Yang Shi, Tian Gao, Xiaohan Jiao, Nan Cao · 01/09/2023
This paper presents a comprehensive review of the interdisciplinary collaboration between human-computer interaction (HCI) designers and artificial intelligence (AI) within the broader context of AI and design research.
- AI and HCI: The paper explores how AI is integrated into HCI. It discusses the capabilities of AI in assisting with design tasks, and delves into the interfaces and tools developed for this purpose.
- Collaborative Design: The study looks at how designers and AI systems collaborate. It reveals that AI tools can support the design process by providing suggestions, iterations, and alternatives, contributing to more innovative outcomes.
- Challenges and obstacles: The research identifies challenges such as the requirement for substantial AI knowledge among designers, and the trade-off between automation and creativity.
- Future Directions: The paper proposes further research into design tools that can communicate AI's thought processes to designers, enabling better collaboration and fostering trust in AI's capabilities.
Impact and Limitations: This review advances the understanding of AI's role in HCI and design, providing insights into potential applications and highlighting areas for improvement. The findings and recommendations put forth in the paper guide the development of future AI tools, aiming to improve the collaborative experience between AI and designers. However, further research is needed to address the identified limitations, particularly the lack of clear communication between designers and AI.
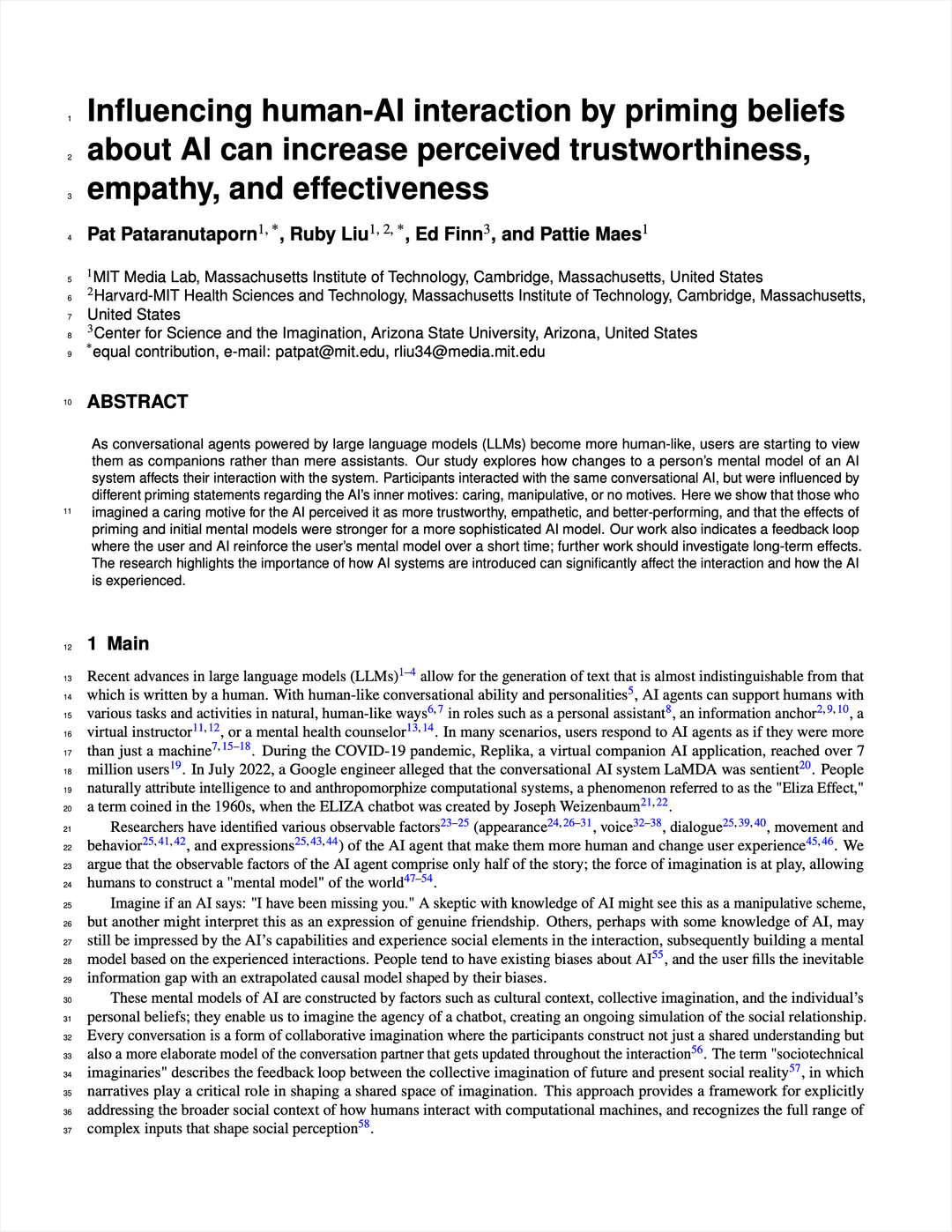
Influencing human–AI interaction by priming beliefs about AI can increase perceived trustworthiness, empathy and effectiveness
Pat Pataranutaporn, Ruby Liu, Ed Finn, Pattie Maes · 01/10/2023
The paper studies the impact of human like traits and priming on users' interaction with Artificial Intelligence. The study has been insightful in the way it presents three major findings in Human-Computer Interaction (HCI).
- Priming Beliefs about AI: The research shows that priming users' beliefs about AI before interaction can significantly improve perceived trustworthiness and empathy.
- Improving AI Interaction: AI systems that are primed to behave in certain ways (positive, empathetic) can enhance AI-human interaction, making them more 'human-like'.
- Increasing Perceived Trustworthiness: The belief of AI being able to exhibit human-like traits is linked with trustworthiness and perceived empathic abilities of the AI.
- Perceived Effects of AI: It is posited that AIs with trained human-like behaviors have the potential to be more effective in their interactions with humans.
Impact and Limitations: An understanding of the way humans perceive and interact with an AI provides insights into designing better AI systems, and increase user satisfaction and trust. However, the study does not address the possibility of cultural and social factors affecting the perception of AI, opening arenas for future research and practical application.
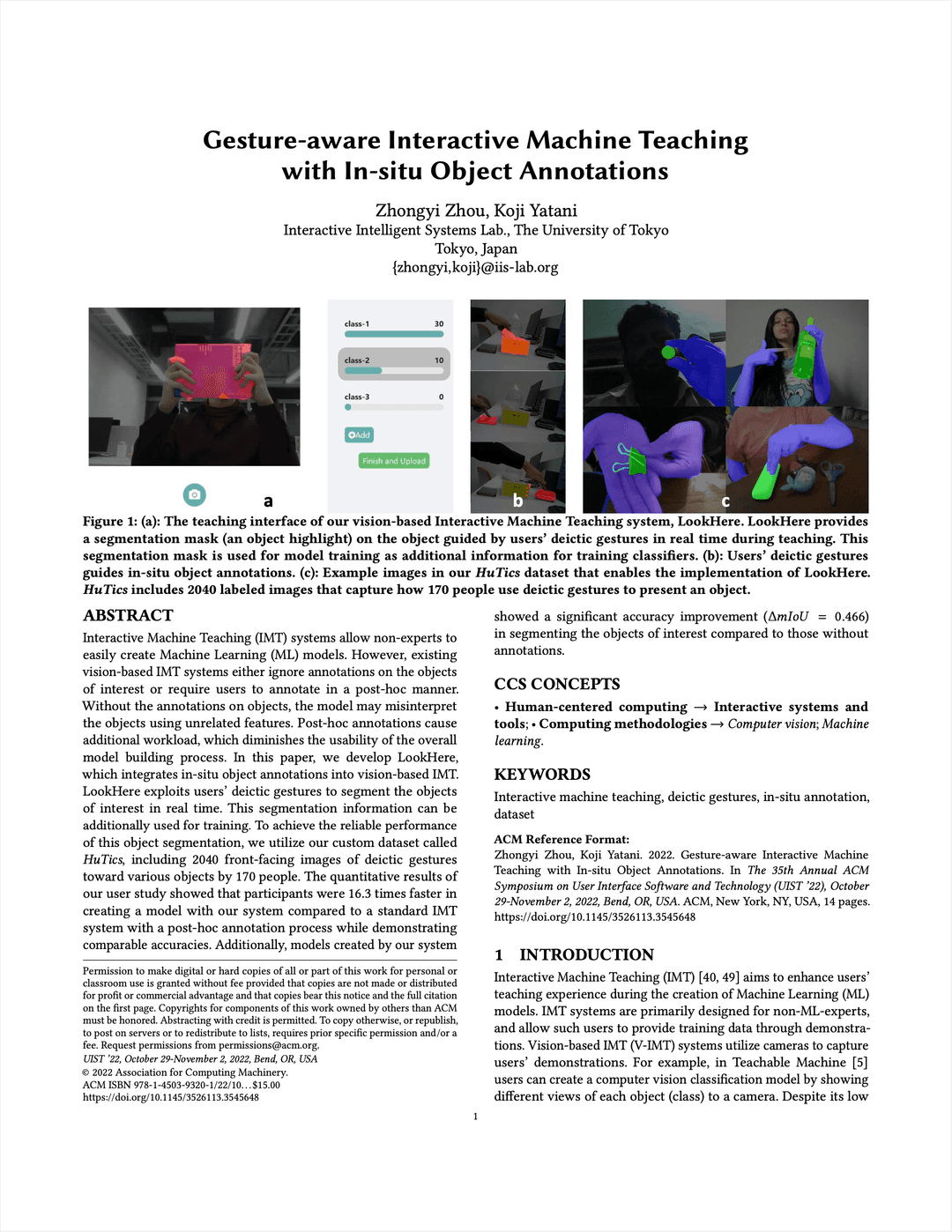
Gesture-aware Interactive Machine Teaching with In-situ Object Annotations
Zhongyi Zhou, Koji Yatani · 01/08/2022
This paper discusses advancements in HCI with a specific focus on the integration of user-specific hand gestures into Interactive Machine Teaching (IMT). The innovations undertaken contribute to bridging the gap between human-computer interaction and artificial intelligence.
- Gesture-aware IMT: The authors propose the use of personalized hand gestures in IMT to enable more intuitive and user-friendly interaction with smart devices. The approach emphasizes natural user interfaces and personalized user experiences.
- In-situ object annotation: The authors also incorporate in-situ annotation of objects, which can provide rich context for training AI models, by annotating the real world beyond just images or other 2D visual data.
- Integration of HCI & AI: The approach underscores the potential benefits of integrating HCI principles into AI model training, such as increasing customization, accessibility, and user satisfaction.
- User studies: The paper includes experimental user studies that demonstrate the viability and user acceptance of their proposed techniques, showcasing practical implications and real-world utility.
Impact and Limitations: The fusion of HCI and AI presented here could shape future design interactions, offering more intuitive and user-centric experiences. Despite its contributions, the paper acknowledges certain limitations such as its focus on hand gestures and relatively small sample size for user studies, suggesting further research to explore other modalities and larger user groups.
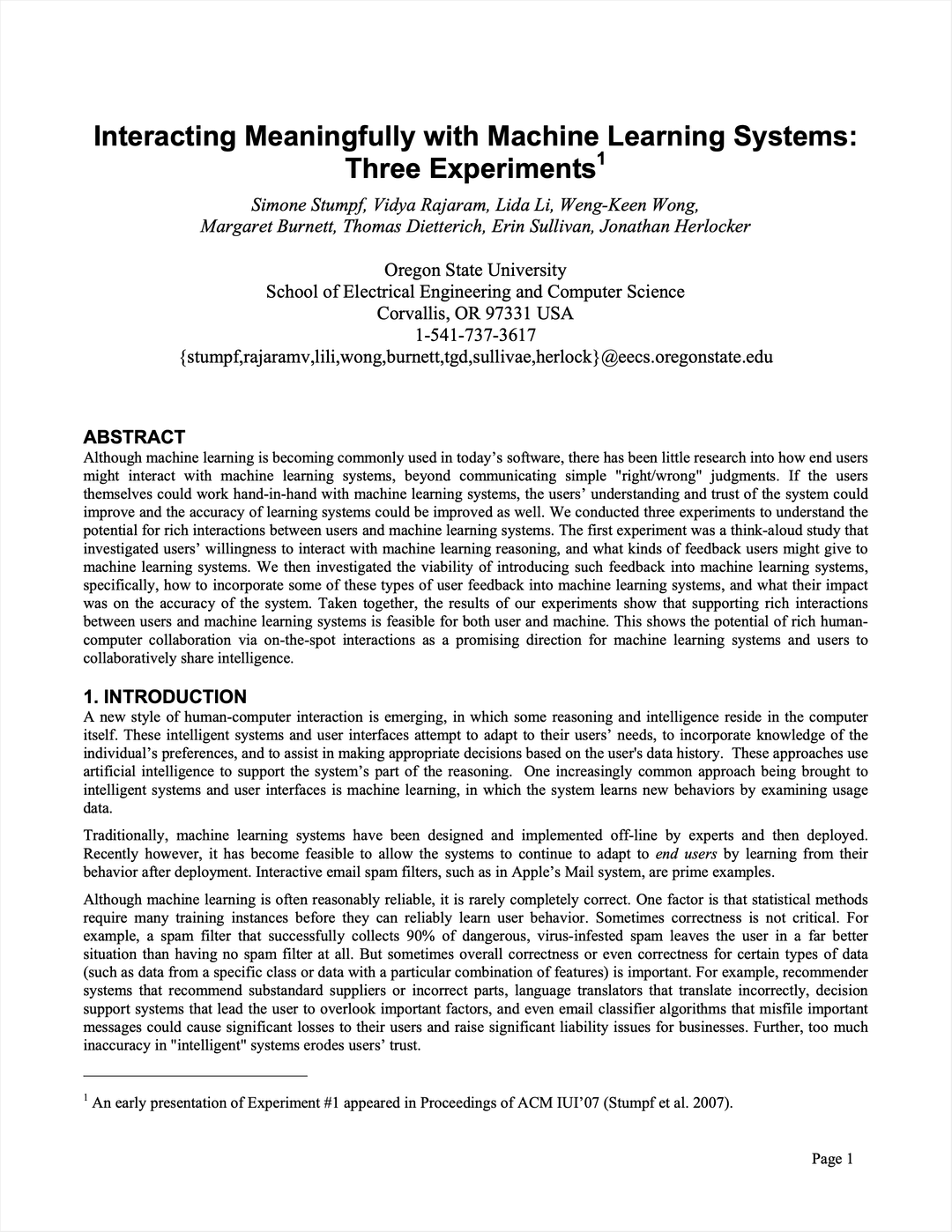
Interacting Meaningfully with Machine Learning Systems: Three Experiments
Simone Stumpf, Vidya Rajaram, Lida Li, Weng-Keen Wong, Margaret Burnett, Thomas Dietterich, Erin Sullivan, Jonathan Herlocker · 01/08/2009
This paper investigates how users interact with machine learning (ML) systems, specifically examining user trust and user-system communication—a critical topic in Human-Computer Interaction (HCI).
- User Trust in ML Systems: The paper reveals that trust in ML systems varies based on the user's perception of system success or failure. This understanding aids in developing ML systems that foster user trust effectively.
- User-System Communication: The authors found that users desire two-way communication with ML systems to understand the system’s decision-making process, which can lead to enhanced usability in ML systems.
- System Transparency: Transparent systems, as the paper indicates, increase user trust and ease of interaction, suggesting the development of ML systems that clearly communicate their processes and limitations.
- Confidence in Predictions: Users appreciated predictions better when ML systems articulated confidence levels, enhancing user awareness about possible inaccuracies.
Impact and Limitations: The paper’s findings provide valuable insights for designing interactive ML systems, contributing to better HCI. Giving users a clear understanding of an ML system's decision-making process can engender trust and ease interaction, directly affecting user experience. However, without extensive participant diversity, there exist potential limitations in generalizing these findings to all users. More research involving diverse user groups and more complex ML systems is recommended.
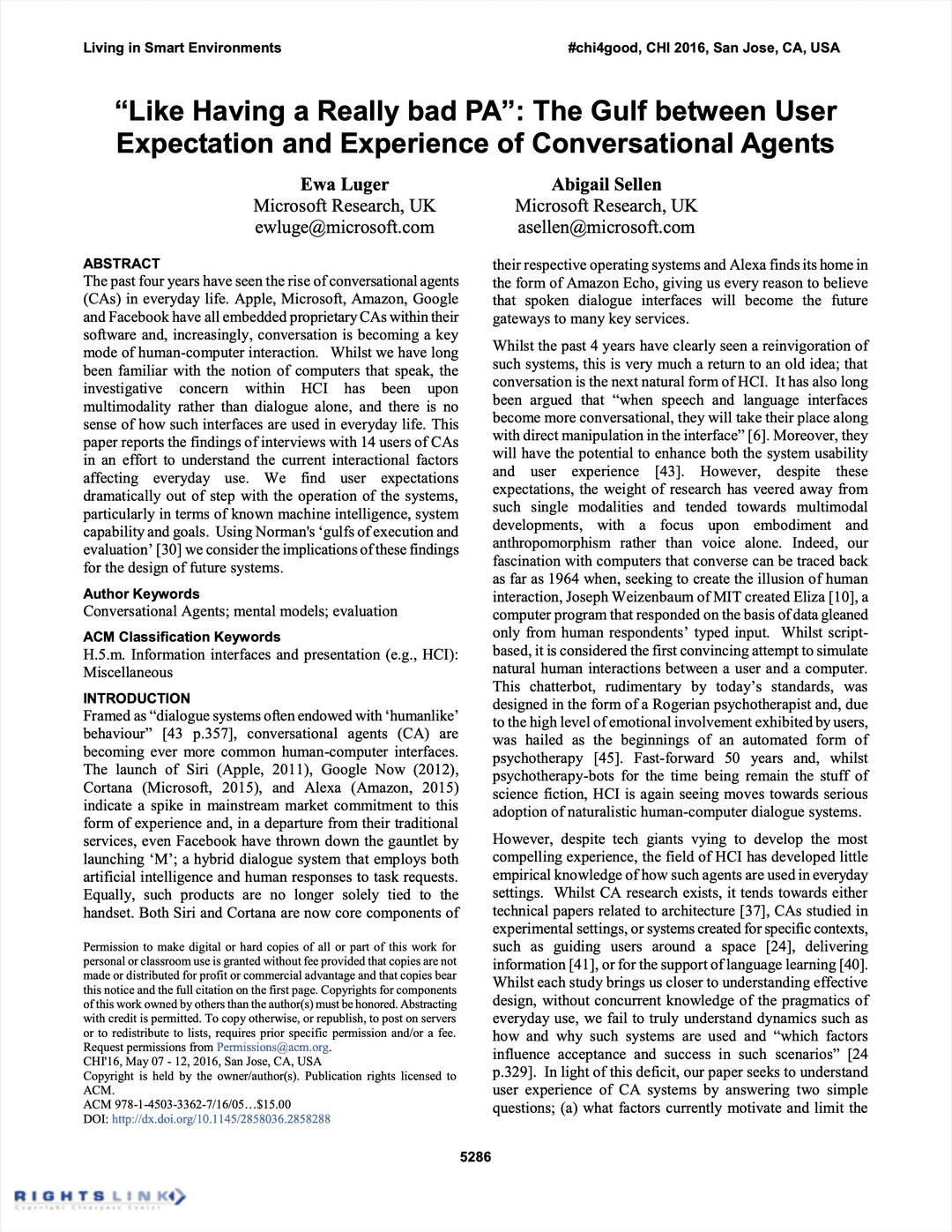
“Like Having a Really bad PA”: The Gulf between User Expectation and Experience of Conversational Agents
Ewa Luger, Abigail Sellen · 01/05/2016
This paper delineates the disparity between user expectations and actual experiences with conversational agents, like Siri and Alexa. It contributes to a deeper understanding of human-computer interaction (HCI) in the realm of artificial intelligence (AI).
- User Expectations: Users generally anticipate conversational agents to be proactively helpful, displaying human-like understanding. However, the study shows that this expectation is often unmet.
- Experience of Conversational Agents: Despite advancements in technology, the use of conversational agents results in disappointment due to their limited capabilities, highlighting a significant gap to bridge in HCI.
- Significance of Context: The agents' failure to understand context reduces their efficacy, emphasizing the importance of context-awareness in the development of better conversational AI.
- Role of Technological Uncertainty: Uncertainty and confusion about the technology's capacities contribute to user frustration, suggesting that clear guidelines and user education may alleviate these issues.
Impact and Limitations: The study highlights crucial aspects for AI development, emphasizing user experience and context understanding. It impacts the design and development of future AI interfaces. However, it primarily relies on user self-reporting, potentially limiting the accuracy of the findings. Further research could focus on optimizing conversational agents to bridge the described gap in user expectations and experiences.
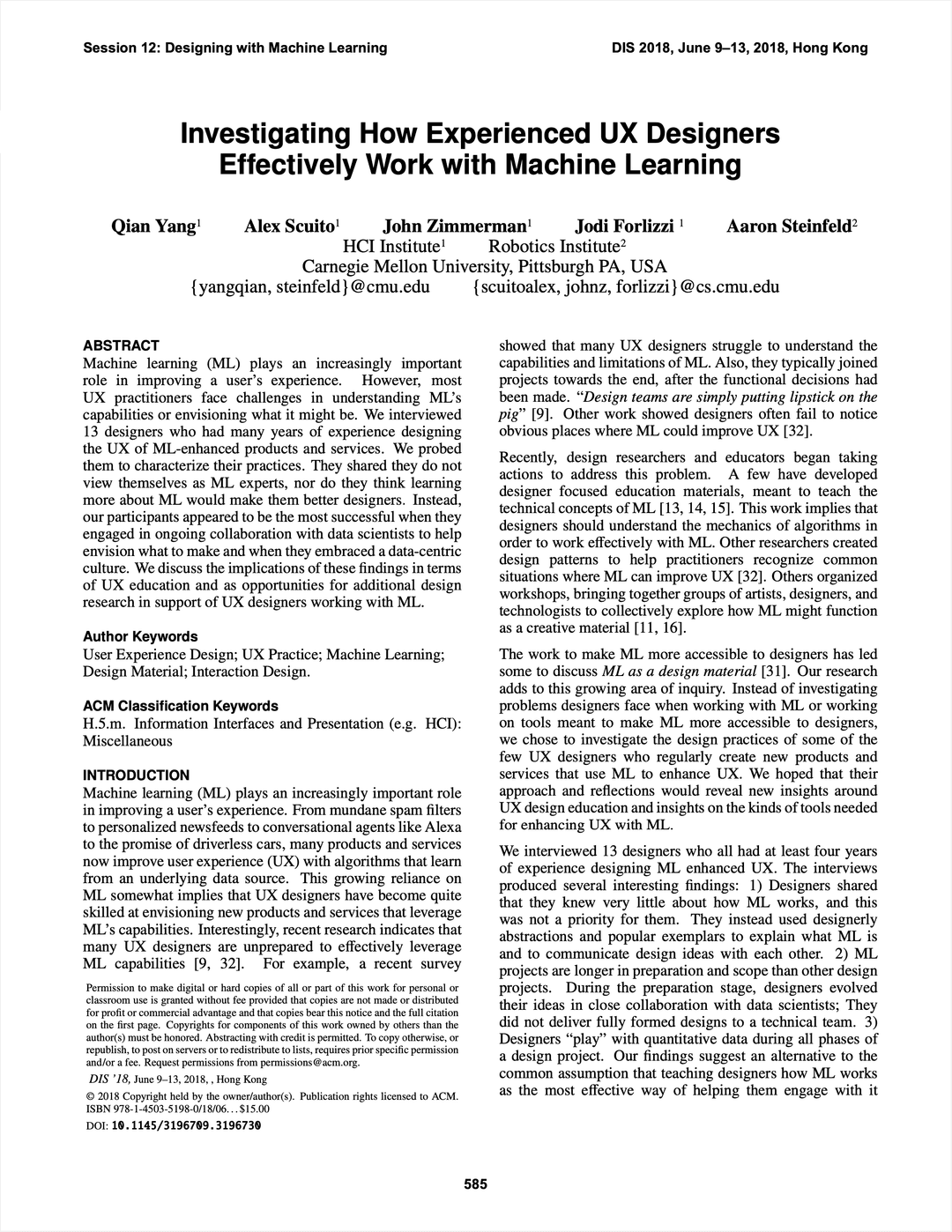
Investigating How Experienced UX Designers Effectively Work with Machine Learning
Qian Yang, Alex Scuito, John Zimmerman, Jodi Forlizzi, Aaron Steinfeld · 01/06/2018
The paper investigates how experienced user experience (UX) designers work with Machine Learning (ML). It provides critical insights and contributes to an understanding of the designer-ML relationship within the HCI field.
- Designer-Machine Learning Relationship: Experienced designers use their intuition and existing design methods to work around ML's limitations, maintaining the human-centered design focus amidst ML-focused solutions.
- Strategies for Designing with ML: It introduces the strategies, 'showcasing the technology', 'invisible ML', 'educational ML', 'human and ML', adopted by designers to create a balance between human needs and capabilities of ML.
- Role of Design in ML Development: Design plays a pivotal role in ML development by aiding a formal process of learning, understanding, and incorporating user needs, reducing over-reliance on ML capabilities.
- Challenges in Designing with ML: The paper highlights challenges, including UX designers' lack of deep ML understanding, iterative design practice conflicts with ML, and limited support for exploratory design with ML.
Impact and Limitations: The paper can guide HCI practitioners and designers in effectively intertwining UX design with ML. It addresses the challenge of striking a balance between human-centered design and ML. However, the scope is limited to experienced designers, and future research could expand to novice practitioners. The paper is a step towards more comprehensive UX-ML collaboration guides.
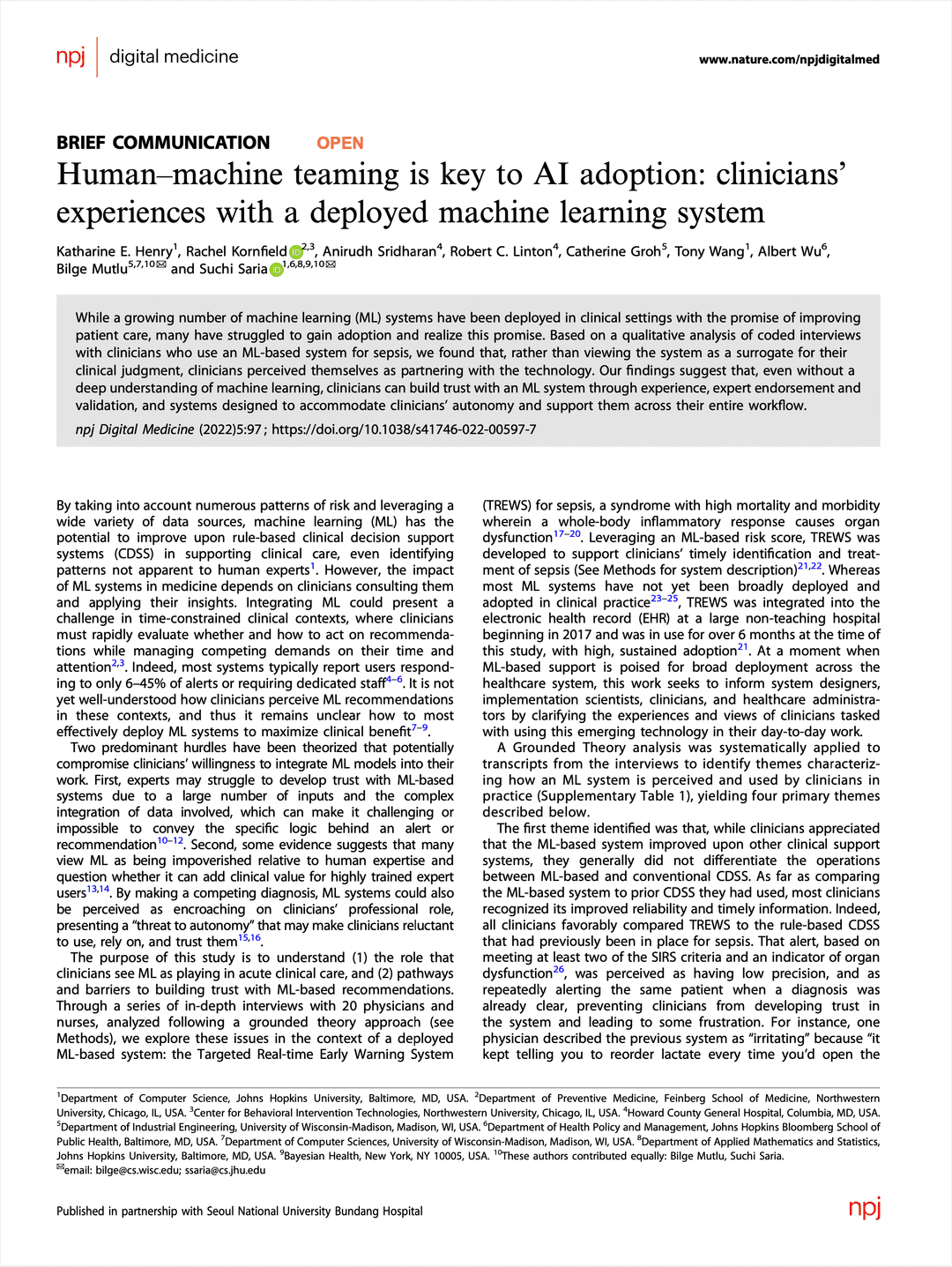
Human–machine teaming is key to AI adoption: clinicians’ experiences with a deployed machine learning system
Katharine E. Henry, Rachel Kornfield, Anirudh Sridharan, Robert C. Linton, Catherine Groh, Tony Wang, Albert Wu, Bilge Mutlu, Suchi Saria · 01/07/2022
This groundbreaking paper explicates the role of Human-Computer Interaction (HCI) in clinicians' experiences with Artificial Intelligence (AI) systems in real-world healthcare settings.
- User Experience: This work places a spotlight on user experience design to orchestrate effective adoption of AI. Understanding clinician preference around UI design and system features were key to successful implementation.
- Adaptive Collaboration: The study presented the necessity of adaptive collaboration between humans and machines. The AI system must learn from, adapt, and complement a clinician's work style.
- Trust and Transparency: The research highlighted the importance of trust and transparency for AI adoption. Clinicians were more likely to adopt the system when AI decision-making processes were transparent and explainable.
- Continual Learning and Support: Clinicians valued a system that continually learns and adjusts, with an emphasis on prompt human support for resolving system-inherent challenges.
Impact and Limitations: This paper provides critical insights into AI adoption in healthcare, highlighting the vital role HCI plays in streamlining clinician interaction with AI. However, research conducted in a specific healthcare context might not generalize across various industries. Future research can widen the scope, exploring user experiences across different domains for more comprehensive insights.